Outsmarting failure. Predictive maintenance powered by machine learning
Since the days of the coal-powered industrial revolution, manufacturing has become machine-dependent. As the fourth industrial revolution approaches, factories can harness the power of machine learning to reduce maintenance costs.
The internet of things (IoT) is nothing new for industry. Worldwide, the number of cellular-enabled factory automation devices reached 270 000 in 2012 worldwide. In 2018 it will rise to a staggering 820 000. Machines are present in every stage of the production process, from assembly to shipment. Although automation makes industry more efficient, with rising complexity it also becomes more vulnerable to breakdowns, as service is both time-consuming and expensive.
Four levels of predictive maintenance
According to PricewaterhouseCoopers, there are four levels of predictive maintenance.
1. | Visual inspection, where the output is entirely based on the inspector’s knowledge and intuition |
2. | Instrument inspection, where conclusions are a combination of the specialist’s experience and the instrument’s read-outs |
3. | Real-time condition monitoring that is based on constant monitoring with IoT and alerts triggered by predefined conditions |
4. | AI-based predictive analytics, where the analysis is performed by self-learning algorithms that continuously tweak themselves to the changing conditions |
As the study indicates, a good number of the companies surveyed by PwC (36%) are now on level 2 while more than a quarter (27%) are on level 1. Only 22% had reached level 3 and 11% level 4, which is basically level 3 on machine learning steroids. The PwC report states that only 3% use no predictive maintenance at all.
Staying on track
According to the PwC data, the rail sector is the most advanced sector of those surveyed with 42% of companies at level 4, compared to 11% overall.
One of the most prominent examples is Infrabel, the state-owned Belgian company, which owns, builds, upgrades and operates a railway network which it makes available to privately-owned transportation companies. The company spends more than a billion euro annually to maintain and develop its infrastructure, which contains over 3 600 kilometers of railway and some 12 000 civil infrastructure works like crossings, bridges, and tunnels. The network is used by 4 200 trains every day, transporting both cargo and passengers.
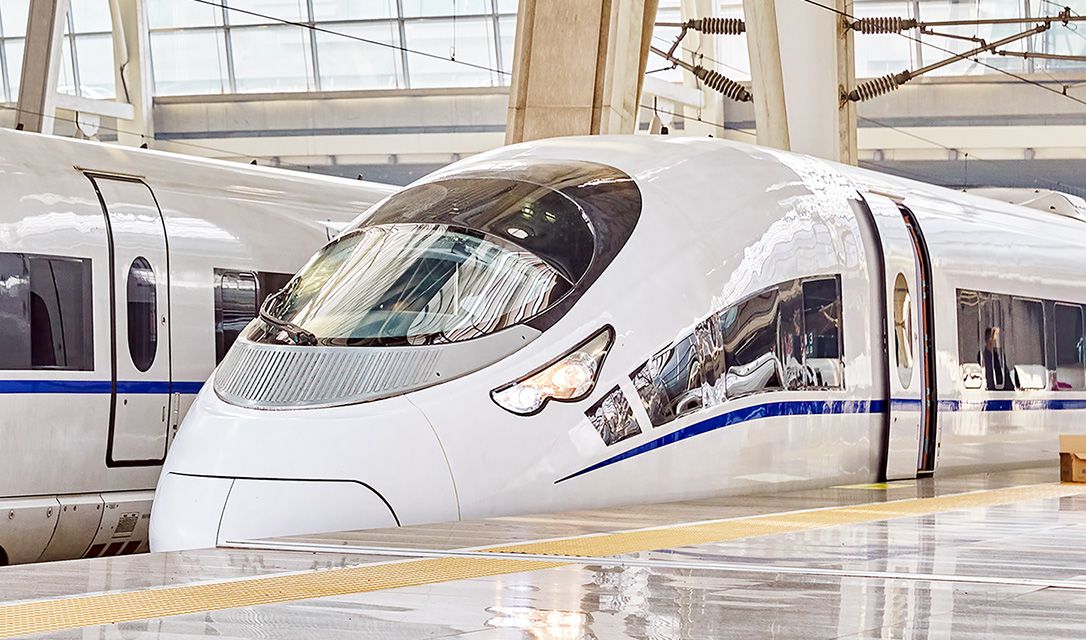
According to the PwC data, the rail sector is the most advanced sector of those surveyed with 42% of companies at level 4, compared to 11% overall.
The company faces both technical and structural challenges. Among them is its aging technical staff, which is shrinking.
At the same time, the density of railroad traffic is increasing – the number of daily passengers has increased by 50% since 2000, reaching 800 000. What’s more, the growing popularity of high-speed trains is exerting ever greater tension on the rails and other infrastructure.
To face these challenges, the company has implemented monitoring tools, such as sensors for monitoring overheating tracks, cameras which inspect the pantographs and meters to detect drifts in power consumption, which usually occur before mechanical failures in switches. All of the data is collected and analyzed by a single tool designed to apply predictive maintenance. Machine learning models are a component of that tool.
As sounding brass
Mueller Industries (Memphis, Tennessee) is a global manufacturer and distributor of copper, brass, aluminum and plastic products. The predictive maintenance solution the company uses is based on sound analysis. Every machine can be characterized by the sound it makes and any change in the tone or the sounds it makes may be a sign of impending malfunction. The analysis of the sound and the vibrations of the machine is done in real-time with the cloud-based machine learning solution that seeks patterns in the data gathered.
Both the amount and the nature of the data collected render it impossible for a human to analyze, but a machine-learning powered AI solution handles it with ease. The devices are able to gather data in ultrasonic and vibration sensors and analyze them in real time. Contrary to experience-based analytics, using the devices requires little-to-no training and can be done on the go.
Endless possibilities
With the power of machine learning enlisted, handling the tremendous amounts of data generated by the sensors in modern factories becomes a much easier task. It allows the company to detect failures before they paralyze the company, thus saving time and money. What’s more, the data that is gathered can be used to further optimize the company’s performance, including by searching for bottlenecks and managing workflows.
That’s why 98% of industrial companies expect to increase efficiency with digital technologies.