Unlocking the Power of Small Language Models with deepsense.ai
Explore how deepsense.ai is helping global leaders like Intel, L’Oréal, and BNP Paribas harness AI with cutting-edge solutions. In this video, we dive into Small Language Models (SLMs) and their role in Retrieval-Augmented Generation (RAG).
Key insights include:
- Benefits of SLMs on edge devices
- RAG pipeline and Android limitations
- Inference speed, memory benchmarks, and demo highlights
Whether you’re implementing AI or optimizing performance, this session offers valuable guidance for your AI journey.
Watch now to see how SLMs can transform your business!
Description
deepsense.ai helps companies implement AI-powered solutions, with the main focus on AI Guidance and AI Implementation Services.
Our commitment and know-how have been appreciated by global clients including Nielsen, L’Oréal, Intel, Nvidia, United Nations, BNP Paribas, Santander, Hitachi, and Brainly.
Wherever you are on your AI journey, we can guide you and help implement projects in Generative AI, Natural Language Processing, Computer Vision, Predictive Analytics, MLOps and Data Engineering. We also deliver training programs to support companies in building AI capabilities in-house.
Errata: in ‘Limited Memory’ slide (07:45) should be Memory in MB (not GB) for each model benchmark.*
Timeline
00:00 Intro
00:32 Small Language Models
01:17 Our Goal – Evaluation of SLMs for RAG
02:00 Benefits of SLMs on edge devices
02:50 Tech Stack
03:48 RAG pipeline
04:46 Android Limitations
05:45 Which Inference Engine for Small LMs?
07:45 Memory Limitations on mobile devices
08:36 SLM inference speed (generation, time to first token)
10:00 Retrieval (timing, memory, mAP)
12:01 Small LMs eval for RAG purpose
15:33 Demo
16:28 Small LMs R&D
Speaker
Kamil Czerski
Senior Team Lead Machine Learning Engineer
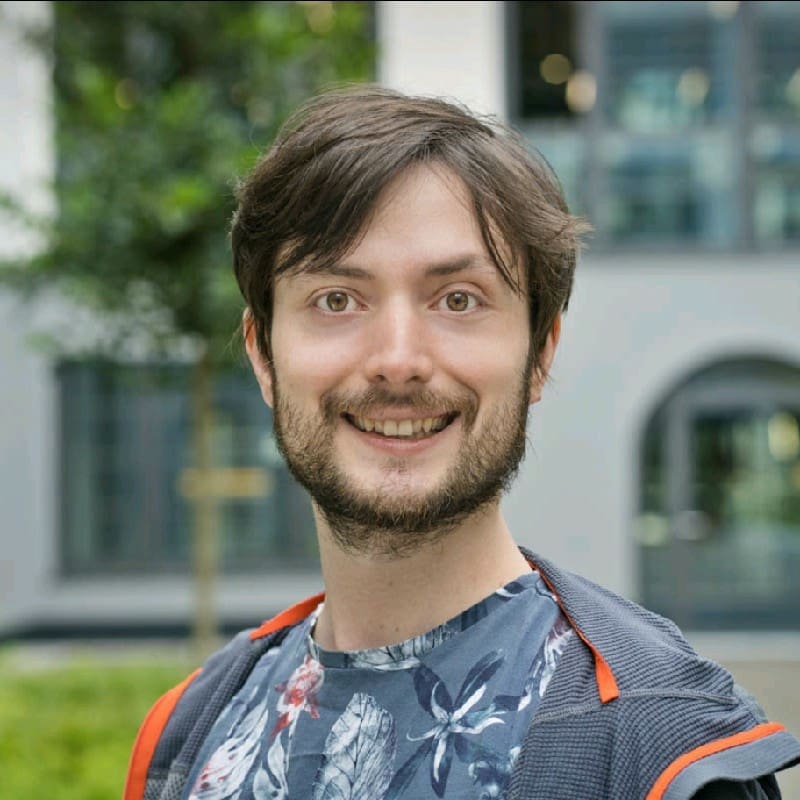