Don’t waste the power. AI supply chain management
Just imagine what your business could do if it recouped 6500 man-hours annually. Or pick up maybe a power supply savings comparable to the electricity used by the entire population of Austin, TX.
This article will describe:
- Why managing modern logistics requires superhuman abilities
- The key challenges in supply chain management
- How much money can be saved when AI is applied to supply chain management
According to Material, Handling and Logistics Magazine, the average US business loses $171,340 per year due to repetitive, mundane and silly tasks like searching for order numbers, processing papers and calculating the value of orders. Yearly, that’s the equivalent of 6,500 man-hours wasted. But they can be reclaimed with modern technology.
Needle in a lorry
The complex flow of goods and services is managed with data and information systems, with the historical orders data, forecasting (for example weather forecasts) and estimations done by the most experienced logistics specialists. Almost every company has tremendous room to boost optimization, which is devilishly complicated.
As a Goldman Sachs analyst quoted by The Economist earlier this year said, there are around 15 septillion (trillion trillion) ways to deliver 25 packages in a lorry or van to their destinations. Given the human brain’s limited perception, sorting out the best of them is no simple task.
A Kaggle competition which the deepsense.ai team took third place in provides a glimpse into how complex logistics challenges can be solved. You can read the story of the algorithm written to manage the delivery of 100,000 christmas gifts from the North Pole here.
Considering the magnitude of the challenge, it should come as no surprise that McKinsey estimates industry outlays on AI for supply chains could reach 1.3-2 trillion dollar.
Where does innovation meet possibility?
Manufacturing is all about striking the right balance between satisfying demand and keeping stock low. The inability to deliver product leads to business losses and results in clients seeking new vendors when their current ones run out of stock. On the other hand, overstocking freezes assets in a warehouse and saps flexibility, as the products in stock–a warehouse full of ice in the middle of Winter–may not suit customers’ demands.
But the supply chain in manufacturing doesn’t only address stock levels. It is also about managing suppliers and shipping, and assigning employees to see to all the dull paperwork and perform repetitive tasks.
Combining computer vision technology, deep learning-powered image recognition and mobile devices, Coca-Cola reduced the work involved in placing orders and managing supplier relationships.
The company trained a neural network to recognize single bottles in a display cooler. With an algorithm that could count the bottles using just a picture taken with an iPad or iPhone, there was no need to send a sales representative to every cooler to inventory the bottles inside.
The information is combined with external data about the weather and past seasonal variations, yielding more precise analysis and predictions. With more than 16 million Coca-Cola coolers spread across the globe, the potential for optimization with AI is clearly enormous.
Power management in supply chain management
With the snowball effect, savings on commodities may provide significant profits for business. Being far from manufacturing, Google proved that putting AI in charge of power supply management may lead to considerable savings. DeepMind analyzed historical data on temperature and the cooling system performance in one of Google’s data centers. Using the data, the system optimized resource management without adding new components or a system redesign.
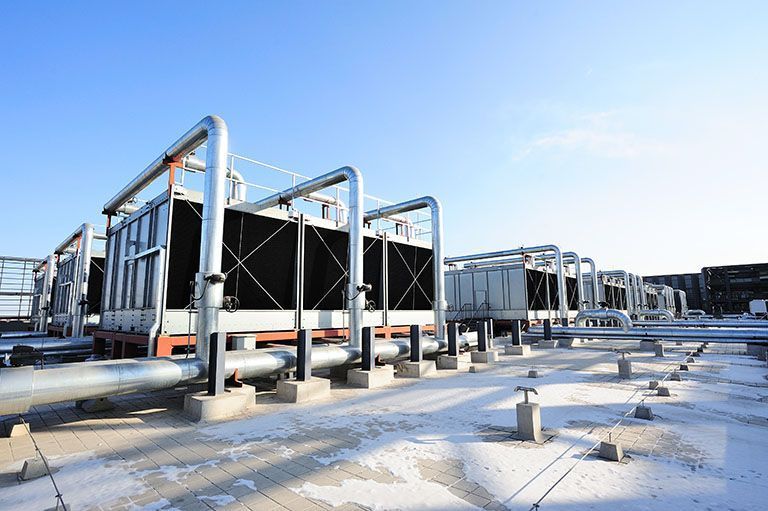
The data center slashed its cooling bill by 40% and overall power consumption by 15%
Most impressively, the data center slashed its cooling bill by 40%, overall power consumption by 15% and the energy it consumed at a rate equivalent to 350,000 US households. Given that there are 2,58 people in the average household, that is slightly less (903 000) than the number of people living in Austin, the capital of Texas (947,890).
Outsmarting logistics
According to another McKinsey study, AI-enhanced supply chain management may boost accuracy by optimizing stock replenishment with 20-50% less forecasting errors. Thanks to AI, transport-related costs are expected to decrease by 5 to 10% and to warehousing and supply an even more impressive 25-40%. Overall inventory reductions of 20-50% are also feasible.
As algorithms efficiently process vast amounts of data with ease, the prospect of further AI-based supply chain development leads to some science-fictionesque visions, including the unassisted anticipation of upcoming orders or autonomous trucks shipping goods on optimal routes across entire continents.
Because AI models are usually tailored to solve some of the biggest challenges in business, an increasing number of companies are recruiting data scientists and building their own AI teams. That task itself may fall under the thorny problems category. Finding a reliable partner that will share its knowledge and support development of an effective AI team may be the way to outsmart not only the competition but all the challenges logistics must deal with today.