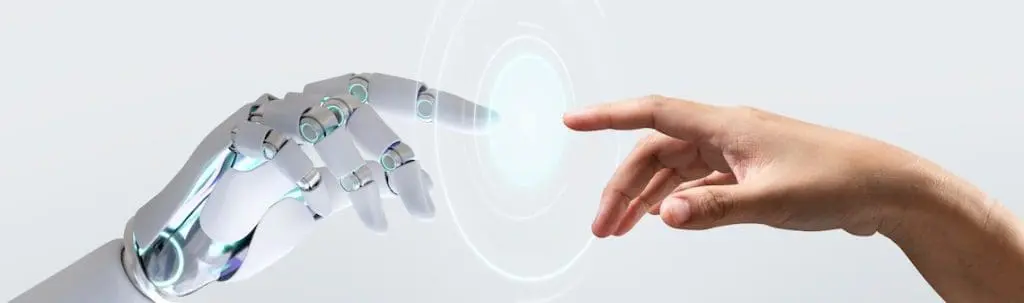
Table of contents
In the world of AI, change is the only constant. The field is evolving at an unprecedented pace, making it extremely challenging for companies and decision-makers to stay ahead of the curve and to keep up with the technical advancements being released day after day. That’s why the ability to adapt and predict future trends for businesses is no longer an option, but a necessity.
Table of contents
Recognizing this high-pressure scenario, six experts from deepsense.ai have taken on the challenge of forecasting the state of AI in 2024. Through rigorous analysis, they spotlight potential developments in six key AI domains, delivering indispensable insights to help savvy leaders prepare for the future.
So, are you ready to uncover what the future holds for AI in 2024? Let’s dive right in!
1. Edge AI – Michał Tadeusiak
In the dynamic realm of artificial intelligence (AI), edge devices are emerging as an enabling force, revolutionizing language communication, shaping the metaverse, and empowering industries. By bringing AI capabilities closer to where data is collected, edge devices enable real-time decision-making, enhanced privacy, and improved scalability.
The field of LLMs is witnessing significant advancements, while companies like Apple, Qualcomm, and Google, by providing platforms like MLX, Snapdragon, and Gemini Nano, respectively, are supporting their adoption closer to users. Meanwhile, the AI community, through initiatives like llama2.c, is diligently working to enable the use of LLMs on edge devices. This on-device capability enhances privacy and scalability, ensuring that sensitive user data remains secure and accessible locally. This effort aims to make intelligent assistants more ubiquitous, offering sophisticated and responsive user interactions on a wide range of devices.
The metaverse is rapidly evolving, enabled by edge AI applications and powerful platforms to support them. Edge AI-powered augmented reality (AR) applications are bridging the gap between the digital and physical worlds, bringing virtual elements into our real-world experiences. Headsets like Apple’s Vision Pro, anticipated for release in 2024, and Meta’s recently launched Quest 3 are spearheading this transformation. These headsets generate real-time overlays of digital information onto the real world, creating immersive and interactive experiences. With the recent developments in the area of 3D scene reconstruction, such as NeRFs and Gaussian Splatting, the future of augmented reality looks brighter than ever before.
While IoT (Internet of Things) and similar edge technologies have been around for some time, edge AI is introducing new possibilities and applications across various industries. In sectors like drones, robotics, and wearables, its influence is marked by the ability to run locally. Edge AI is particularly effective in predictive maintenance, utilizing sensor data for proactive upkeep, reducing repair costs and downtime. Retail is also experiencing a transformation with edge AI, where automated checkout systems and AR-enhanced in-store navigation are redefining the shopping experience. The automotive industry stands out in its adoption of edge AI, especially in developing autonomous vehicles. A prime example is Tesla’s Full Self-Driving (FSD) beta program, which leverages deep learning models on edge devices for advanced autonomous driving features. This innovation highlights edge AI’s capability in executing real-time, complex tasks, setting the stage for the widespread use of self-driving cars in the near future.
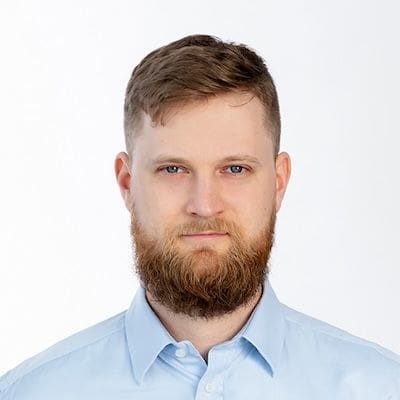
Edge AI is rapidly becoming a foundational technology across multiple sectors. Its transformative applications in language, the metaverse, and industries demonstrate its versatility and ability to enable significant advancements. 2024 will see the rise of edge AI applications which are poised to play an even more integral role in shaping our digital interactions, enhancing operational efficiencies, and empowering users with intelligent and personalized experiences. Michał Tadeusiak, Director of AI
2. Large Language Models – Mateusz Wosiński
Unless you have been locked in a closet for about a year, you no doubt know that we are living in the era of Large Language Models (LLMs). Although the first such solutions were developed as far back as 2019 (e.g. GPT-2 from OpenAI), the release of the famous ChatGPT in November 2022 was arguably the biggest breakthrough. At that moment, the revolution began, and almost everybody got extremely hyped about this technology.
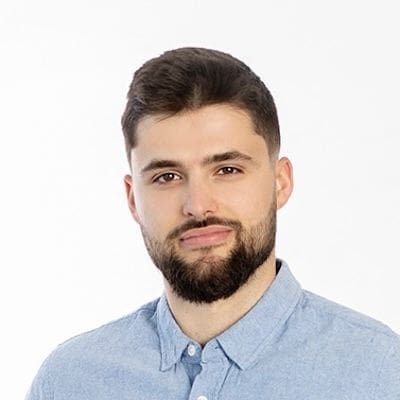
Next year is expected to be a year of transitioning LLM-based applications from research to production. Those who identify the opportunities first will benefit the most. Mateusz Wosiński, Senior Machine Learning Engineer
Since the debut of our all-new favorite web tool, we have seen several models attempting to further improve its outstanding quality and mitigate the most crucial drawbacks – hallucinations, cost and data privacy. All the tech giants have joined the race, each taking a different approach:
- Meta released an open-source family of models called LLaMA,
- Google delivered PaLM and LaMDA models which are accessible via a ChatGPT-like assistant called Bard,
- Lastly, Microsoft, or to be more exact OpenAI, which received a multi-year, multi-billion dollar investment from that giant of the industry, refined its previous models with a multi-modal GPT-4, capable of understanding not only text, but also images.
Apart from that, we have observed numerous examples from academia (an open-source Alpaca model, which is basically a fine-tuned LLaMA, from Stanford AI lab being the most notable one) and newly emerging companies (e.g., Anthropic, which released a new model Claude, designed to be “helpful, harmless and honest”).
But what does it all mean for business? An endless stream of possibilities! LLMs are such a revolutionary technology that the majority of companies have not yet figured out the possible use-cases. However, there certainly are plenty of them. At deepsense.ai, we have already delivered a couple of projects leveraging such solutions, including the Frontline Worker Assistant, which provides specific instructions based on internal knowledge bases, or Interactive Document Explorer, which strongly accelerates the process of understanding complex PDFs. What’s more, we collaborate closely with LangChain, the leading framework for creating LLM-powered applications. Our team was responsible for some of its most important features concerning data privacy and app security. As a result, deepsense.ai was awarded the prestigious title of official LangChain partner. And we are just getting started!
Next year will undoubtedly surprise us all with further impressive model advancements, but I expect it to be specifically a year of implementing LLMs into full-scale production. And those who miss the wave, may fall far behind.
3. 3D scene reconstruction – Konrad Czarnota
3D scene reconstruction based on camera images is currently a focal point in the AI community. The ability to digitize real-world items and bring them to the virtual world has become reality.
The current trend sees a shift from special devices with dedicated hardware, such as LIDAR, to basically any smartphone capable of recording videos. Recent developments of NeRFs (Neural Radiance Fields) and Gaussian Splatting methods have streamlined the entire process. As a consequence, the required GPU memory and training times have significantly decreased, making these algorithms more accessible.
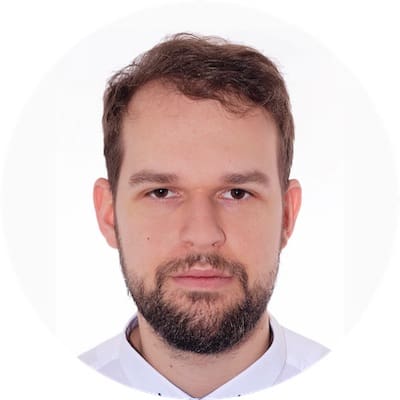
In the upcoming years, scenes created using either Gaussian Splatting or NeRFs are likely to achieve mainstream popularity. Their potential to seamlessly integrate real-world scenes into the virtual realm promises a thrilling business opportunity that’s hard to ignore. Konrad Czarnota, Senior Data Scientist
These advancements have led to the successful application of 3D scene reconstruction in numerous industries. Let’s take a closer look at a few of them. E-commerce businesses, for instance, can now generate 3D views of their products much faster and, importantly, at a much lower cost. Recent advancements have also brought a significant change to the special effects industry, enabling the creation of complex scene representations of real-world buildings, all based on drone-captured footage. Companies that manage large stadiums can now produce views from each individual seat automatically, a feature that dramatically enhances ticket sales. The entire entertainment industry is gearing up for the possible incoming personalization opportunities these advancements can offer for users wishing to import physical items into the virtual world.
Here at deepsense.ai, we’re at the forefront of innovation. We’ve been hands-on, experimenting with the latest breakthroughs in 3D scene reconstruction, even taking our own office as a testing ground, which has led to some interesting conclusions. In our exploration, we discovered that Gaussian Splatting offers superior visual effects but grapples with certain challenges in early stage development, particularly the lack of support for some tools. On the other hand, NeRFs have evolved considerably over the past few years, providing a stable and well-supported set of tools. However, they could occasionally produce more visible artifacts, such as mist-like or blurred areas. I strongly believe that in 2024 Gaussian Splatting will quickly surpass NeRFs to become the most sought-after solution for novel view synthesis. Meanwhile, NeRFs themselves may hone their focus on highly specialized use-cases, such as few-shot scene generation derived from just a handful of images. As we move forward, we anticipate a steady increase in scenes created using these techniques. In the coming years, they’ll likely go mainstream. Their potential to seamlessly integrate real-world scenes into the virtual realm promises a thrilling opportunity that’s hard to ignore.
4. Diffusion models – Maciej Domagała
Without a doubt, diffusion-based models have taken the computer vision-related GenAI scene by storm. These models are stripped of the limitations one might experience with typical GAN-based and VAE-based applications. As of 2023, there are two strong observable trends propelling each other forward. OpenAI’s series of groundbreaking DALL·E models are often referred to as a trendsetter in terms of quality for text-to-image solutions. On the other hand, we can observe the virtually limitless application of diffusion models, thanks to the publication of the open-source powerhouse Stable Diffusion.
The global availability of the latter has resulted in a much faster development of task-specific architectures for, e.g., inpainting or video-from-image rendering. Transfer learning and domain adaptation are thriving thanks to sharing services such as HuggingFace or Civitai. This is a huge benefit for companies as it brings them several steps closer to incorporating many of the latest models directly into their workflows. The recent surge in the development of multi-modal methods is clearly visible in the domain adaptation field. New state-of-the-art structures, such as ControlNet, are utilizing numerous types of inputs (both text and image-related) to generate customized output.
As the quality of the models rises, we expect to see more automation happening in the near future to make these convoluted architectures even more accessible to businesses. This trend has already begun, as, for instance, the newest DALL·E 3 is supported natively by ChatGPT, which – putting aside the usage-related costs – makes it a viable option for organizations trying to maximize the impact of innovation on their businesses. Beyond the general accessibility provided by diffusion models, there is also the generation speed aspect. This year’s SDXL Turbo enables high-quality single-step generation which allows for near-instant image generation. All of these new ideas consistently make the field interesting and ever more exciting. At deepsense.ai, we like to keep up with the leading advancements and we are certain that 2024 will bring a lot of interesting research!
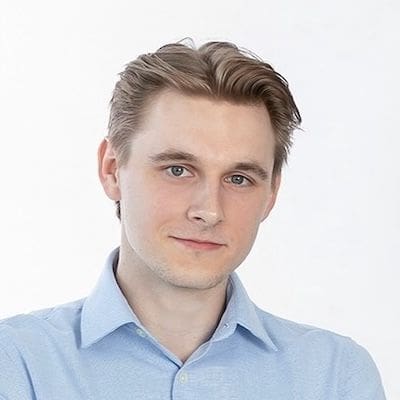
In 2024, we expect even greater abstraction of the current architectures, allowing users to enhance seamless finetuning of custom domain models. Open-source diffusion-based solutions (among others) will benefit from the global multi-modality trend, which will help to unleash their potential. Maciej Domagała, Senior Machine Learning Engineer
5. LLMOps – Mateusz Hordyński
The rapid rise in popularity of Large Language Models (LLMs) has undeniably revolutionized our approach towards machine learning project development. These models have enabled us to blueprint and bring to life product ideas at unprecedented speed. Prompt engineering has pushed back the heavy lifting, such as data preparation or model training, to the later stages of development, allowing teams to focus earlier on innovative and creative aspects of their projects. Essentially, this shift has empowered teams that were previously unable to use AI in their products due to technical or resource limitations to do so. This has given rise to countless PoCs, demos and AI startups over the last year. However, we’re still in the early days of LLM adoption – getting them to do meaningful work is yet to happen. That is precisely what’s going to happen in the LLMOps community in 2024.
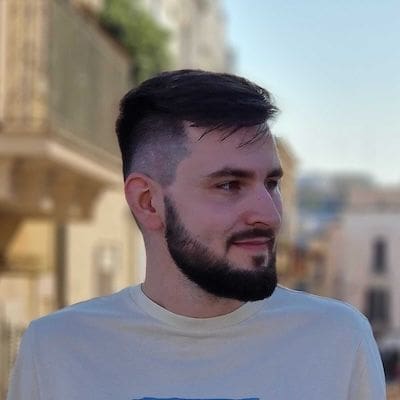
In 2024, a transition awaits LLMOps as we move from LLM-powered PoCs to production-grade systems, enabling companies to create reliable and profitable products. To achieve this, we need the emergence of more performant inference serving, observability, and security tools. Additionally, tools to distill LLM knowledge into smaller, more efficient specialist models are yet to be developed. Mateusz Hordyński, Technical Leader
In the upcoming months, inference serving in LLMOps will see significant enhancements. The core trend in this area will focus on achieving scalable and efficient inference deployments while maintaining high model performance. Given that LLMs are very demanding and often require huge amounts of memory and storage, it is crucial to optimize usage of those resources. The popularity of more advanced quantization methods is expected to rise, aiming to reduce overall model sizes and, consequently, lower latency. There is potential for popular inference serving libraries to further optimize model performance by leveraging more sophisticated attention algorithms, tensor, and data parallelism methods. Another cost-saving method involves serving multiple fine-tuned specialist adapters to a single foundational model as a base, thereby minimizing overhead.
Observability and security will also be key aspects of LLMOps trends in 2024. Increased visibility of model behavior will become more critical to ensure the robustness and reliability of AI systems. The development of tools that provide transparency into how LLMs make decisions, monitoring model performance in real-time, and identifying any drifts or anomalies in model predictions will be emphasized more strongly. Furthermore, we will likely see a surge in the adoption of LLM-related security tools, and observable systems will enable us to analyze traffic against popular attack surfaces, such as prompt injections.
Lastly, a very interesting concept is to replace large generalist models with smaller, more specialized versions for specific tasks. Techniques like LLM distillation – training smaller models using LLMs – may significantly increase in popularity. This area also can greatly benefit from more advanced tooling – for supervising the learning process, gathering labels, and sourcing reasoning data from LLMs.
6. Coding Agents – Maks Operlejn
AI tools are revolutionizing the programming landscape, enhancing efficiency and quality in software development. According to a study, GitHub Copilot (a tool for autocomplete code) has sped up the work of software developers by as much as 55%. Programmers themselves also believe that code quality has improved as well.
In 2023,LLMs like GPT-4 have transformed how we interact with coding resources. It’s undeniable that GPT has at least partially replaced the good old Stack Overflow in the coding process, if not completely. But what if AI could do more than just help with code snippets? What if it could build entire code repositories with only objective specification? That’s where Coding Agents come into play:
- The user specifies the goal (e.g., “create a system to manage the company’s inventory”), adds the required technical specification (such as “use the following technologies: […]”) and provides any necessary information (like “users should be able to create an account via activation email”).
- AI-driven assistants will plan, prioritize, and generate full-scale code in line with specified goals and technical requirements. They can craft code, conduct on-the-fly testing, and refine with real-time ‘Reflection’ mechanisms, while still allowing human collaboration through feedback and manual code enhancements.
Despite their potential, Coding Agents face two main challenges:
- Need for Current Data: While GPT-4 is excellent for code generation, its training data only goes up to 2021 – a sizable gap in the ever-evolving tech world. This leads to concerns about outdated code and incompatibilities.
- Limited Prompt Length: A code repository could encompass hundreds of files. Conveying comprehensive context to ensure that AI-generated code integrates seamlessly with existing systems is a significant hurdle.
Both problems are addressed in various ways by users (for example by using RAG systems), but companies are also identifying and fixing models’ weaknesses. Not long ago, a new version of GPT-4 Turbo came out which increases the context size and includes data up to April 2023.
Beyond the realm of Coding Agents that operate using mainly LLMs, there is an additional frontier where graphical prototypes are being transformed into code. A key innovator in this field is Figma, which is already conducting trials on converting User Interface (UI) design into workable code via AI. This approach bridges the gap between designers and developers, thus promoting a more integrated and collaborative workflow.
Coding Agents are still at the early stages, with a lot of growth expected before they’re ready for widespread business use. In 2023, they might seem like fun toys to play with. But looking ahead to 2024, there’s a strong possibility that they will become more practical and reliable tools for developers. Let’s ponder a future where AI can take over a variety of roles – planning projects like a Product Owner (and putting them in Jira), breaking down tasks like an Analyst (describing Jira issues), and even managing code submissions and testing (via GitHub). While this concept might seem as though it belongs in the realm of science fiction and people are just experimenting with prototypes now, one trend is clear: software developers are going to rely more on AI for coding assistance, and will spend more of their time reviewing and approving the AI’s work.
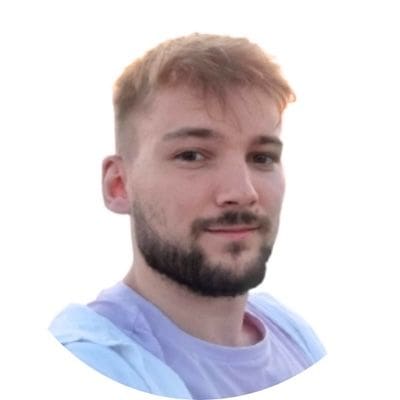
AI tools can significantly accelerate developers’ work and have a direct effect on business success. The focus in programming is sometimes shifting more towards “reviewing” code rather than “writing” it from scratch. While fully autonomous Coding Agents are still the wave of the future, keeping up to date with current AI-based tool advancements is essential to avoid missing out on key opportunities. Maks Operlejn, Machine Learning Engineer
It’s widely recognized now that AI-assisted tools are essential for modern developers. Those who don’t adopt these tools risk falling behind, as their productivity may dwindle. The reality is, with the aid of AI, businesses can significantly accelerate their software development process. Here at deepsense.ai, keeping up to date with AI technology is in our DNA, and these tools are integral to our daily operations. This article delves into existing agent coding solutions, and excitingly, a follow-up article showcases our venture into developing a proprietary agent – we invite you to explore our findings and innovations!
Summary
As we navigate this rapidly unfolding technological revolution, the challenge lies not only in understanding and keeping up with the rapid advancements, but also in strategically harnessing these developments to drive innovation, growth, and competitiveness. As AI continues to break boundaries and redefine possibilities, it’s an exciting time to be part of this transformative journey.
The future of AI may seem like venturing into the unknown, but with expert insights, we can better prepare for what’s to come in order to avoid being left behind by competitors. So let’s embrace the forthcoming changes and boldly step into the innovative, AI-driven world of 2024!