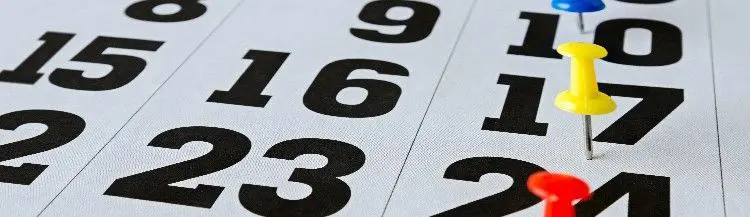
Table of contents
Table of contents
In the latest Seahorse release we introduced the scheduling of Spark jobs. We will show you how to use it to regularly collect data and send reports generated from that data via email.
We would like to generate some graphs from this data every now and then and do it without any repeated effort.
For the historical weather data, we create a data source representing a file stored locally within Seahorse. Initially, it only contains a header and one row – current data.
Now we can create our first Spark job which reads both data sources, concatenates them and writes back to the “Historical weather data” data source. In this way, there will be a new data row in “Historical weather data” every time the workflow is run.
There is one more step for us to do – this Spark job needs to be scheduled. It is run every half an hour and after every scheduled job execution an email is sent.
In the email there is a link to that workflow, where we are able to see node reports from the execution.
In a notebook, first we transform the data a little:
Finally, we can set up a schedule for our report. It is similar to the previous one, only sent less often– we send a report every day in the evening. After each execution two emails are sent – one, as previously, with a link to the executed workflow and the second one containing a notebook execution result.
… and graphs that are in the executed notebook:
Use case
Let’s say that we have a local meteo station and the data from this station is uploaded automatically to Google Sheets. That is, it contains only one row (i.e. row number 2) of data which always contains current weather characteristics.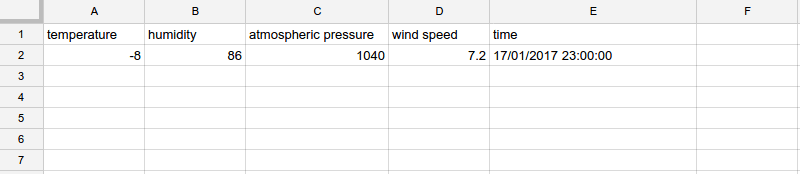
Solution
Collecting data
The first workflow that we create collects a snapshot of live data and appends it to a historical data set. It will be run at regular intervals. Let’s start by creating two data sources in Seahorse. The first data source represents the Google Spreadsheet with live-updated weather data: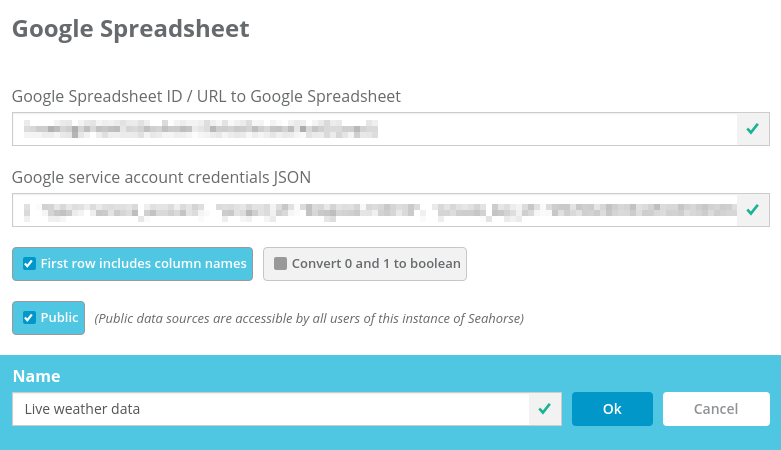
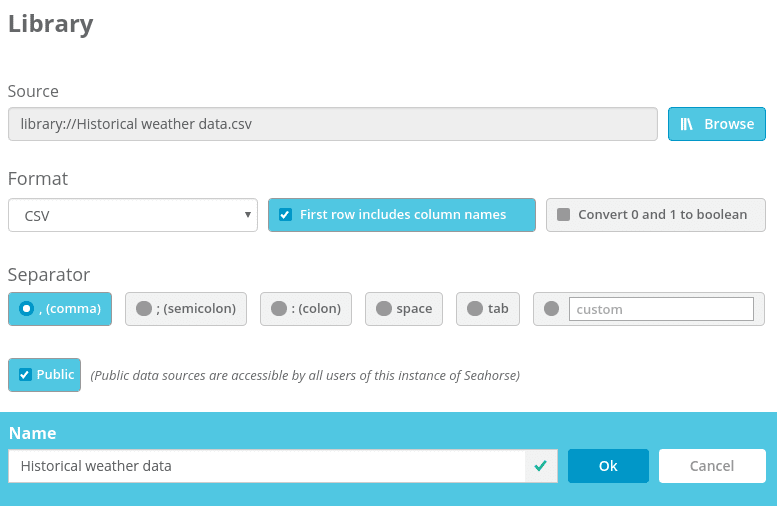
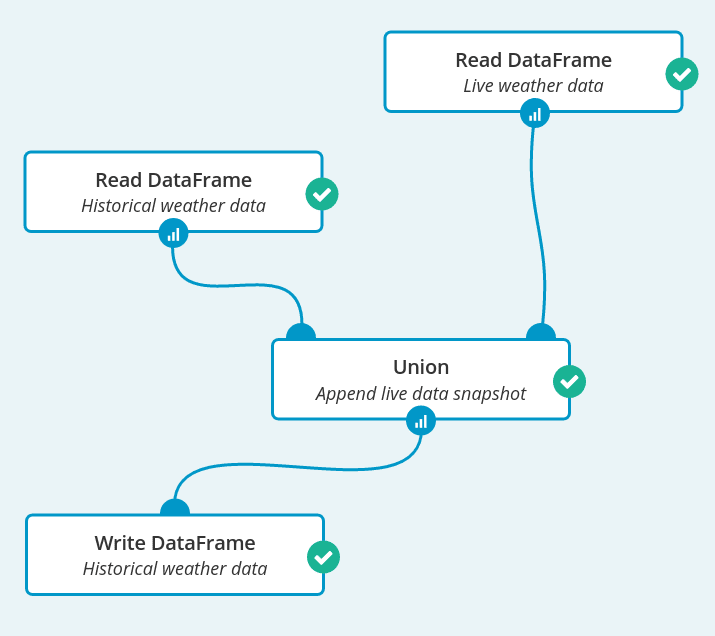
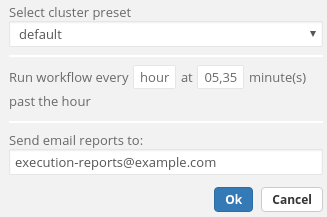
Sending a weather report
The next workflow is very simple – it consists of a Read DataFrame operation and a Python Notebook. It is done separately from collecting the data because we want to send reports at a much lower rate.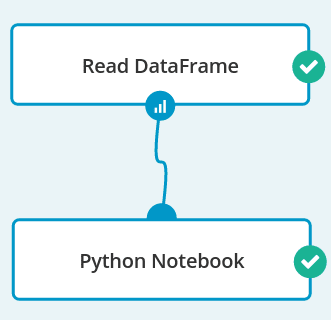
import pandas import numpy import matplotlib import matplotlib.pyplot as plt %matplotlib inline data = dataframe().toPandas() data['time'] = pandas.to_datetime(data['time']) data = data.sort('time')Next, we prepare a function that will be used to line-plot a time series of weather characteristics like temperature, air pressure and humidity:
def plot(col_to_plot): ax = plt.axes() ax.spines["top"].set_visible(False) ax.spines["right"].set_visible(False) ax.get_xaxis().tick_bottom() ax.get_yaxis().tick_left() y_formatter = matplotlib.ticker.ScalarFormatter(useOffset=False) ax.yaxis.set_major_formatter(y_formatter) plt.locator_params(nbins=5, axes='x') plt.locator_params(nbins=5, axes='y') plt.yticks(fontsize=14) plt.xticks(fontsize=14) plt.plot(data['time'], data[col_to_plot]) plt.gcf().autofmt_xdate() plt.show()After editing the notebook, we set up its parameters so that reports are sent to our email address after each run.
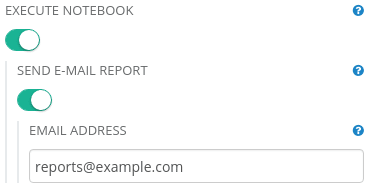
Result
After some time, we have enough data to plot it. Here is a sample from the “Historical weather data” data source…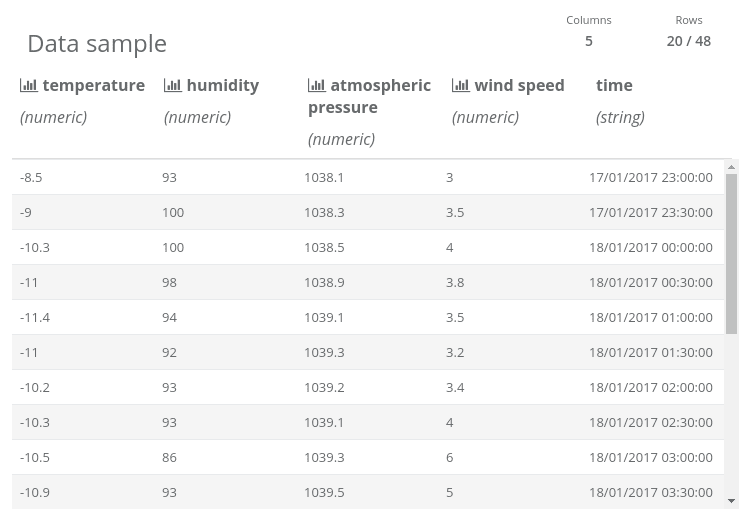
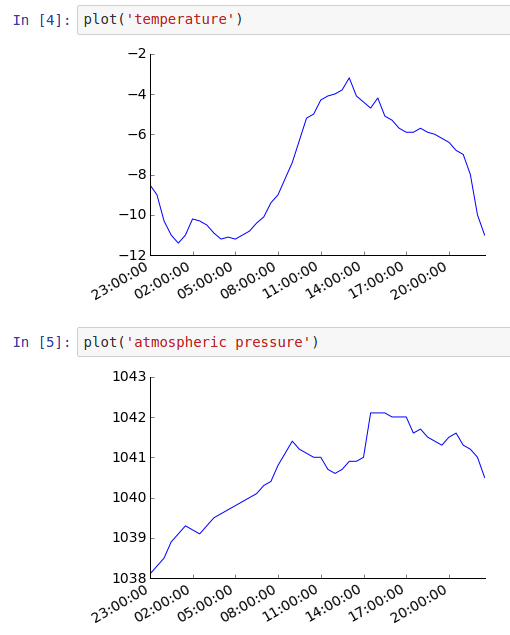