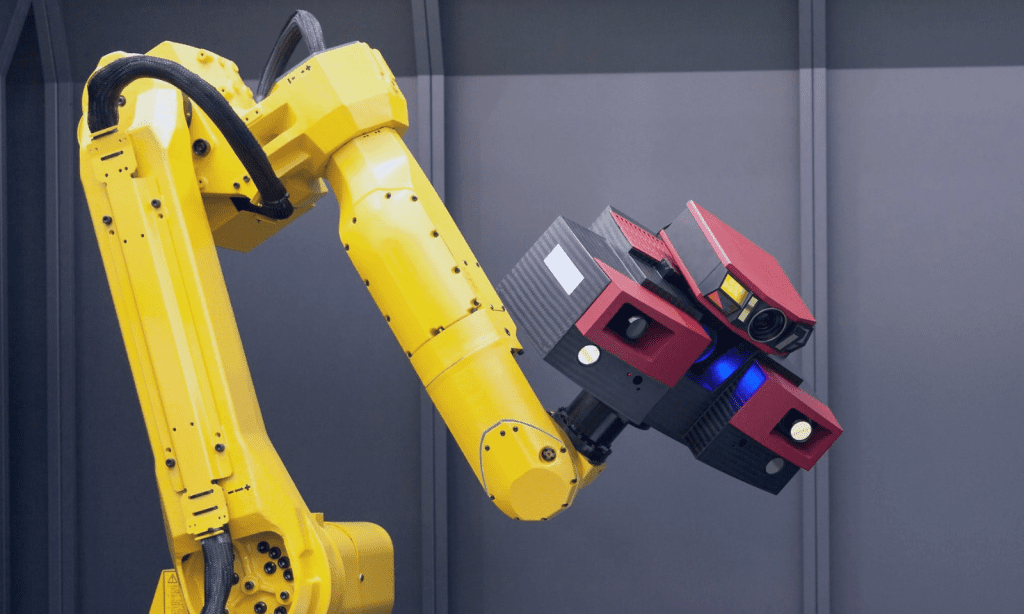
The successful quantization process enabled our client to integrate state-of-the-art computer vision models into their end devices, with only a 6% increase in error compared to the baseline models.
Meet our client
Client:
Industry:
Market:
Technology:
Client’s Challenge
A camera appliance manufacturer aimed to enhance their products with embedded AI. To implement sufficiently powerful deep neural networks, they needed to optimize the models to fit within the limited storage and computational resources of the end devices. The project’s KPI set a strict limit, allowing no more than a 30% increase in errors compared to the baseline float32 model.
Our Solution
We employed advanced neural network quantization techniques, including Post-Training Quantization (PTQ) and Quantization-Aware Training (QAT). These methods reduced the numerical precision of the networks from float32 to int8.
Client’s Benefits
The successful quantization process enabled our client to integrate state-of-the-art computer vision models into their end devices, with only a 6% increase in error compared to the baseline models. This enhancement significantly bolstered their smart-camera value proposition, solidifying their competitive advantage in the market.