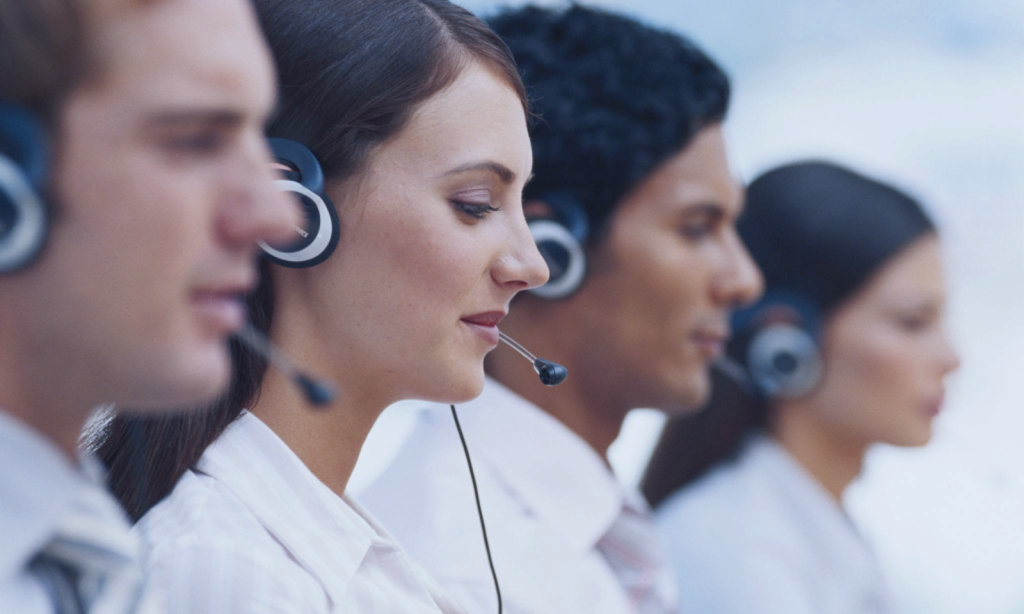
We delivered a 27.4% reduction in MAPE compared to the previous business-as-usual approach, significantly improving forecast reliability.
Meet our client
Client:
Industry:
Market:
Technology:
Client’s Challenge
A leading European telecom operator needed to scale predictive models to forecast daily call center volume—often tens of thousands of calls. Accurate forecasts, especially for customers near contract end, were crucial for staffing and churn reduction. Key challenges included inconsistent customer segmentation across departments and fragmented data sources, making segment-specific modeling difficult.
Our Solution
We implemented automated ML models using Vertex AI pipelines within the client’s GCP setup, leveraging Prophet and Singular Spectrum Analysis for accurate, interpretable forecasts. We also delivered Tableau dashboards with conservative, business-friendly outputs to support decision-making.
Client’s Benefits
We delivered a 27.4% reduction in MAPE compared to the previous business-as-usual approach, significantly improving forecast reliability. These predictions now inform strategic decisions across commercial, finance, and marketing teams, helping to better allocate call center staffing and identify opportunities for investment that reduce inbound calls. While recently launched, the new system is replacing older models and building trust among internal teams.