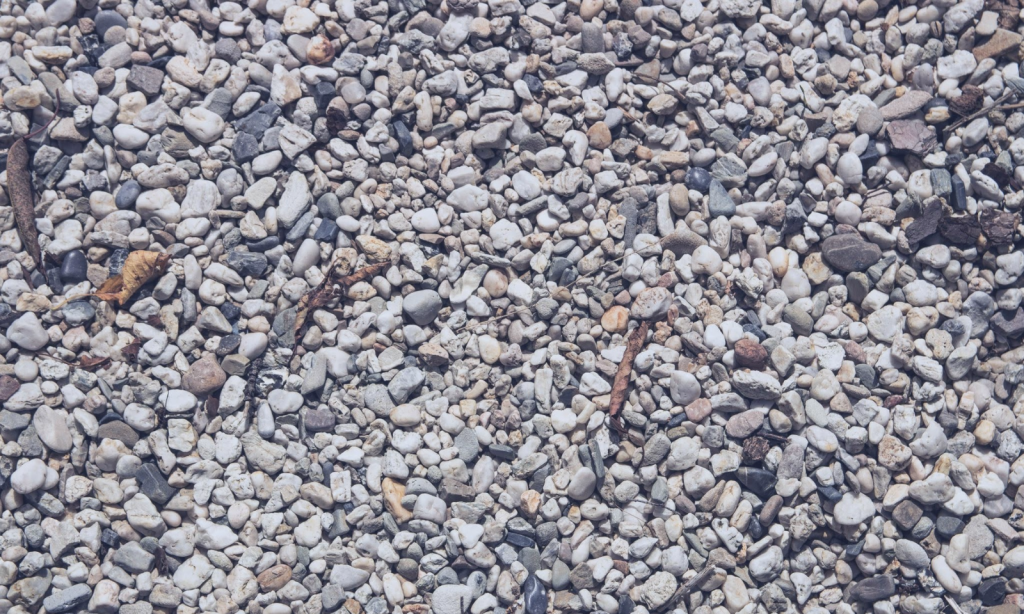
Our solution streamlined the segmentation and classification process, achieving a 65% accuracy rate at IoU 0.75 while reducing the need for manual labeling.
Meet our client
Client:
Industry:
Market:
Technology:
Client’s Challenge
The client required an accurate method to identify and segment various impervious surfaces (e.g., cobblestone, pavement tiles, gravel) from aerial imagery to support urban planning. Traditional supervised learning was unsuitable due to the lack of labeled data and the high variability in surface appearances.
Our Solution
We developed an unsupervised image segmentation pipeline using the Segment Anything Model (SAM) combined with data preprocessing, clustering, and post-processing techniques. This approach enabled effective segmentation without relying on extensive labeled datasets.
Client’s Benefits
Our solution streamlined the segmentation and classification process, achieving a 65% accuracy rate at IoU 0.75 while reducing the need for manual labeling. Scalable and adaptable to large datasets and diverse urban environments, it offers an efficient tool for urban planning and infrastructure management.