Powering up demand forecasting with machine learning
Demand forecasting is a field of predictive analytics, that aims to predict the demand of customers. It is done by analyzing statistical data and looking for patterns and correlations. Machine learning takes the practice to a higher level.
According to McKinsey study, AI-enhanced supply chain management may lead to improved accuracy by reducing forecasting errors by 20-50%.
Why demand forecasting is essential
There is always a context surrounding customer behavior. It may be an upcoming holiday, the weather or a recent event. As real product demand varies, businesses may face two challenges:
- Income and profit loss when a product is out of stock or a service is unavailable
- Cash tied up in stock or
- The reduced margins that come with getting it out of warehouses
According to the Harvard Business Review, history is littered with missed demand forecasts that came with great losses for the business. To name a few of the most important:
- In 1974, the U.S. energy sector made plans to double power generation due to a forecast calling for 7% annual growth in demand. But during the 1975-1985 period, growth faltered, coming in at a mere 2%. While many projects were canceled, the industry was heavily hit and consumer prices rose.
- In 1983 and 1984, the PC market was forecast to explode and see 27-28 million units installed by 1988. As the story goes, by 1986 only 15 million units had been shipped and many PC manufacturers had gone out of business.
Considering the essential role of accurate demand forecasts, it is no surprise that an ARC global market study cited by Forbes predicts that demand applications will account for just under a third of the $2 billion supply planning suites market.
Human-powered demand forecasting methods
Demand is predicted using both qualitative and quantitative methods. In the most basic form, it is done by leveraging the experience of the seller or collective brainstorming in the company. Another popular way is to ask the customers. Conducting market surveys is one of the simplest methods of demand forecasting and may be applied in all industries, no matter the size or market segment. Other common methods include delphi forecasting method that relies on a panel of experts answering questions or employing game theory an leveraging.
As companies are relying more and more on the data they collect, it is no surprise that traditional, human-based methods are being replaced by data-powered, automated systems. Using big data demand prediction is enabling a wide range of companies to leverage machine learning models in data exploration and extrapolation. If there is enough data to train a model, it is almost certain to outperform human data analysts and researchers. For proof of that, have a look at Google’s Sunroof project, which makes more accurate predictions of a roof’s sun exposure than any human analyst could do.
Demand prediction done right – case studies
Modern demand forecasting techniques go far beyond simple historical data analysis. When Netflix’s launched the design process for its series House of Cards, the company analyzed the most replayed parts of shows and films featuring Kevin Spacey, the show’s main star.
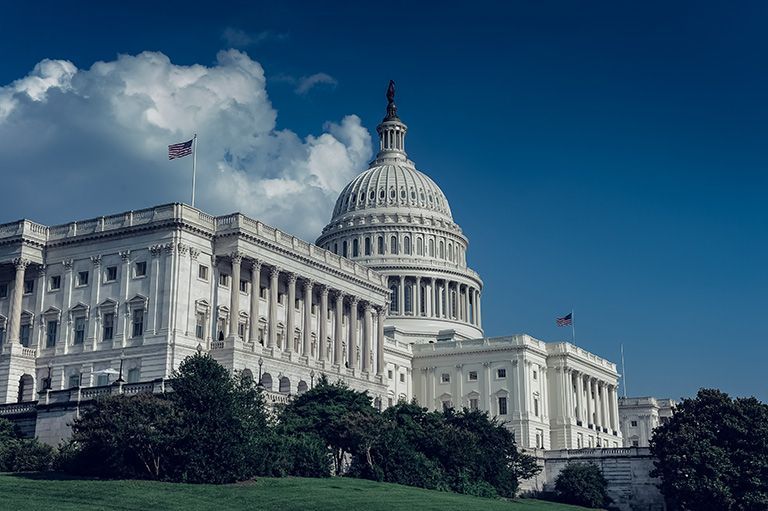
When Netflix’s launched the design process for its series House of Cards, the company analyzed the most replayed parts of shows and films featuring Kevin Spacey, the show’s main star.
This helped it analyze what the target group was looking for more of. The data mining proved invaluable as the show’s second episode peaked at more than 4 million viewers. And according to the “Esquire”, the absence of Kevin Spacey in the last season “haunts the production” and the show is not the same anymore without the key actor.
Good demand forecasting can be a literal life-saver. In the UK, patients rely on the National Health Service’s (NHS) Blood & Transplant department, which consists of five manufacturing sites and 15 stock holding units. The department is responsible for transporting blood between hospitals, and managing both the donations and deliveries. The NHS’ previous system was relied heavily on manual data management, but it didn’t have the technology to analyze the data. Introducing a data mining-based system enabled the NHS to forecast demand by blood type and quantities it needed. With its new system, the NHS is able to prevent shortages by refreshing stock level readings every 30 minutes and connecting the information from various sources. Participating hospitals reported a 30% reduction in overstock waste and a 20% cut to delivery costs.
Summary
As demand forecasting is statistics-heavy and data-rich, it is ripe for the application of machine learning algorithms. The automation of data flows helps to manage logistics and find new ways to optimize an organization’s performance.