Region of interest pooling in TensorFlow – example
In the previous post we explained what region of interest pooling (RoI pooling for short) is. In this one, we present an example of applying RoI pooling in TensorFlow. We base it on our custom RoI pooling TensorFlow operation. We also use Neptune as a support in our experiment performance tracking.
Example overview
Our goal is to detect cars in the images. We’d like to construct a network that is able to automatically draw a box around every car.
In our example we deal with car images from the Pascal VOC 2007 dataset. For simplicity we choose only cars not marked as truncated.
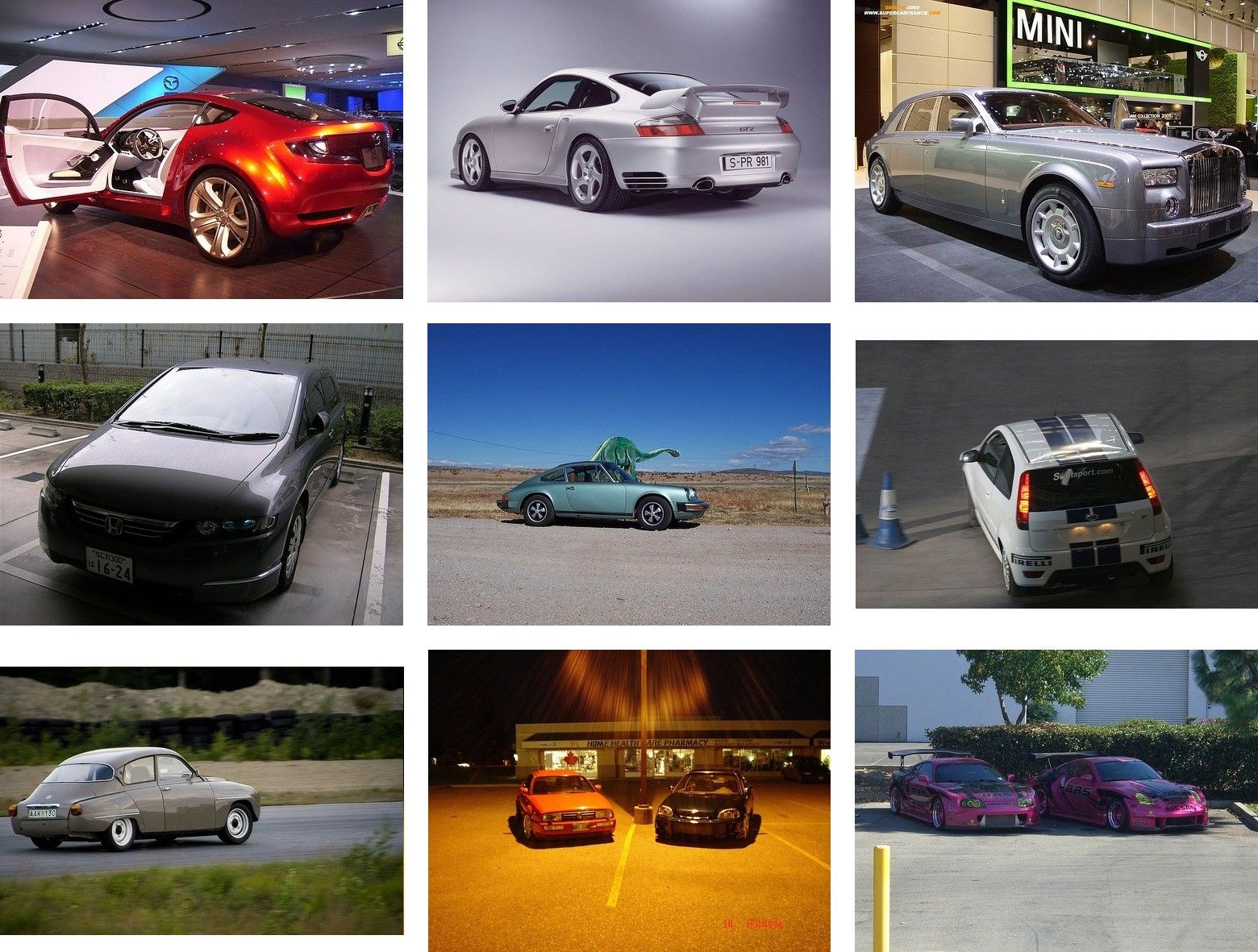
Neptune
We manage our experiment using Neptune. It’s a pretty handy tool:
- We track the tuning in real time. Especially, we preview the currently estimated bounding boxes.
- We can change model hyperparameters on the fly.
- We can easily integrate Neptune with TensorFlow and get all the charts, graphs and summary objects from the TensorFlow graph.
- We store the executed experiments in an aesthetic list.
Network architecture
In our example we use the Fast R-CNN architecture.
The network has two inputs:
- Batch of images
- Batch of potential bounding boxes – RoI proposals
In the Fast R-CNN model RoI proposals are generated via an external algorithm, for example selective search. In our example, we take ground truth bounding boxes from the Pascal annotations and generate more negative bounding boxes ourselves.
The network has two outputs:
- Batch of RoI proposals not classified as background (with corrected coordinates)
- Probabilities that RoI proposals consist of objects of the consecutive categories
The network consists of three main parts:
- Deep convolutional neural network
- Input: images
- Output: feature map
We use the popular VGG16 network pretrained on the ImageNet dataset.
- RoI pooling layer
- Input: feature map, RoI proposals resized to a feature map
- Output: max-pooled RoI proposals
- Fully connected layer with RoI features
- Input: max-pooled RoI proposals
- Output: corrected RoI proposals, probabilities
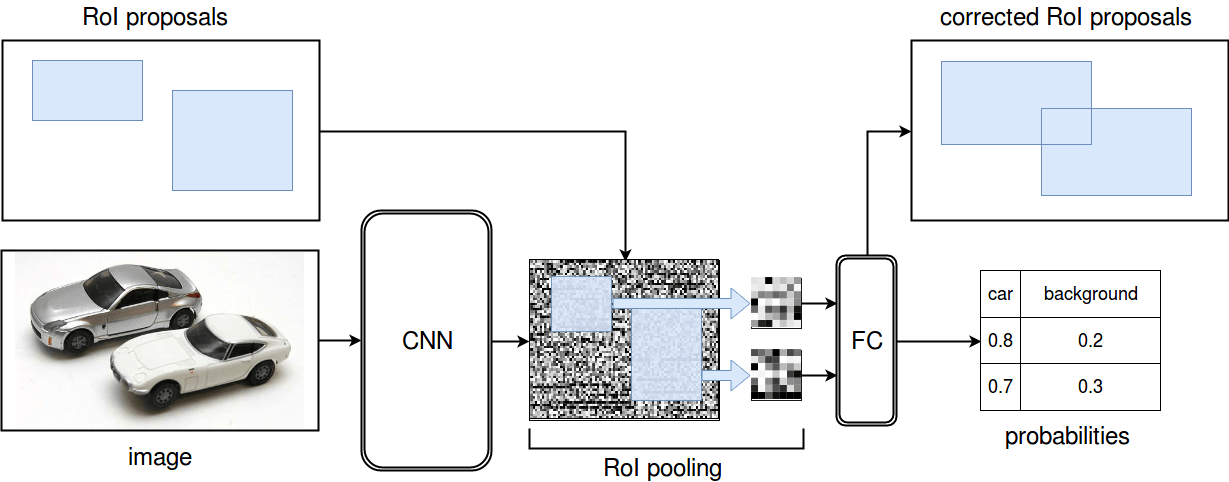
We note that our detection task can be also solved with the Faster R-CNN architecture, which works significantly faster :). However, the implementation of Faster R-CNN requires much more code to write, so we chose the simpler Fast R-CNN.
Loss function
We tune the network to minimize the loss given by
\(loss = frac 1nsum_{i=1}^n frac 1{k_i} sum_{j=1}^{k_i} loss_{ij}\)
where:
- \(n\) is a number of images in a batch,
- \(k_i\) is a number of RoI proposals for the image \(i\),
- \(loss_{ij}\) is a loss for the RoI proposal \(j\) for the image \(i\).
For a single RoI proposal, \(loss_{ij}\) is the sum of the classification and regression loss, where:
- classification loss is the common cross entropy,
- regression loss is a smooth L1 distance between the rescaled coordinates of a RoI proposal and the ground-truth box. The regression loss is computed if the ground-truth box is not categorized as background, otherwise it’s defined as 0.
Implementation details
Prerequisites
To run the code we provide, you need the following software:
- CUDA 8,
- TensorFlow 1.0 with GPU support,
- our custom RoI pooling TensorFlow operation,
- OpenCV,
- Neptune (version 1.5): apply for our Early Adopters Program or try it immediately with Neptune Go.
Repository
You can download our code from our GitHub repository. It consists of two folders with the following content:
File | Purpose |
---|---|
code | |
main.py | The script to execute. |
fast_rcnn.py | Builds the TensorFlow graph. |
trainer.py | Preprocesses data and trains the network. |
neptune_handler.py | Contains Neptune utilities. |
config.yaml | Neptune configuration file. |
get_data.py | Downloads images from Pascal VOC 2007 dataset |
data | |
vgg16-20160129.tfmodel.torrent | References to weights of the pretrained network. |
Description
When we run main.py , the script trainer.py first restores the VGG16 network with the pretrained weights. Then it adds the RoI pooling layer and the fully connected layer. Finally, it begins tuning the entire network with use of provided images and RoI proposals. It also sends information to Neptune, so we can track the tuning progress in real time.
After cloning the repository, please download the file vgg16-20160129.tfmodel referred to by the torrent file vgg16-20160129.tfmodel.torrent and save it in the data directory. Also, please run the script get_data.py to download needed images:
python get_data.py
Let’s test our RoI pooling in TensorFlow!
We run the script main.py from the code folder by typing:
neptune run -- --im_folder $PWD/../data/images --roidb $PWD/../data/roidb --pretrained_path $PWD/../data/vgg16-20160129.tfmodel
If we want to also use a non-default learning rate value or the number of epochs, we can add:
--learning_rate 1e-03 --num_epochs 200
to the command at the end.
After a while, we can start observing the tuning progress in Neptune:
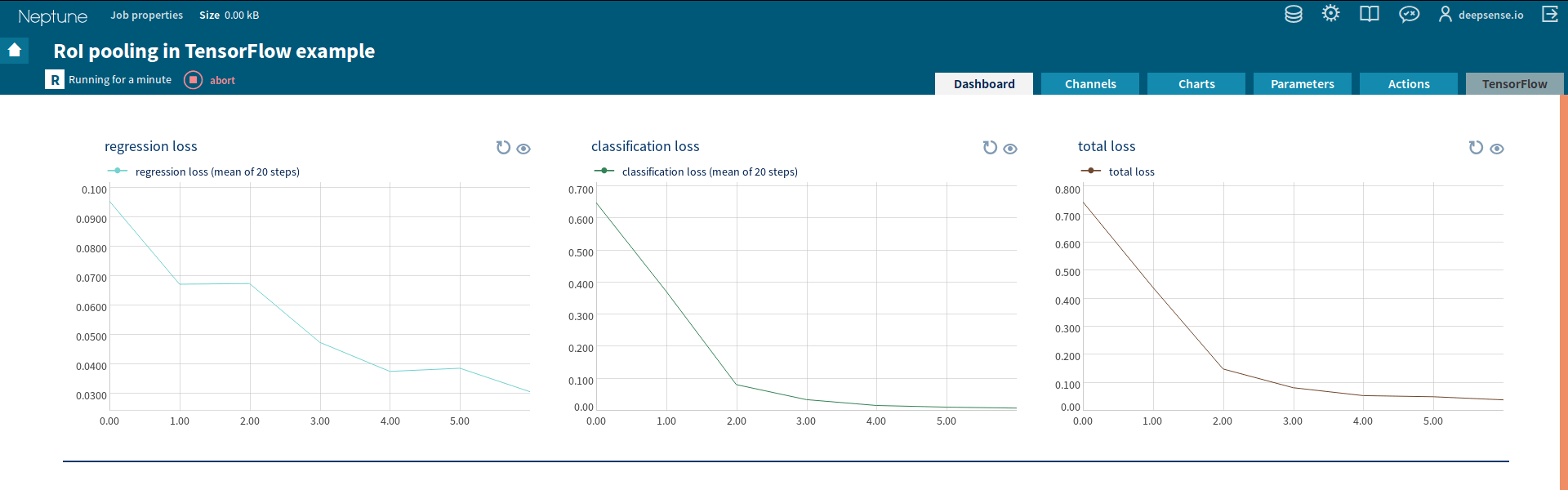
Moreover, we can display the RoIs fitted to the cars by our network. We could just load all the processed images, but this procedure would take much of resources. That’s why we decided to activate this feature by a simple Neptune action.
To do that, we can go to the Actions tab and click ‘RUN’ to start sending the images.
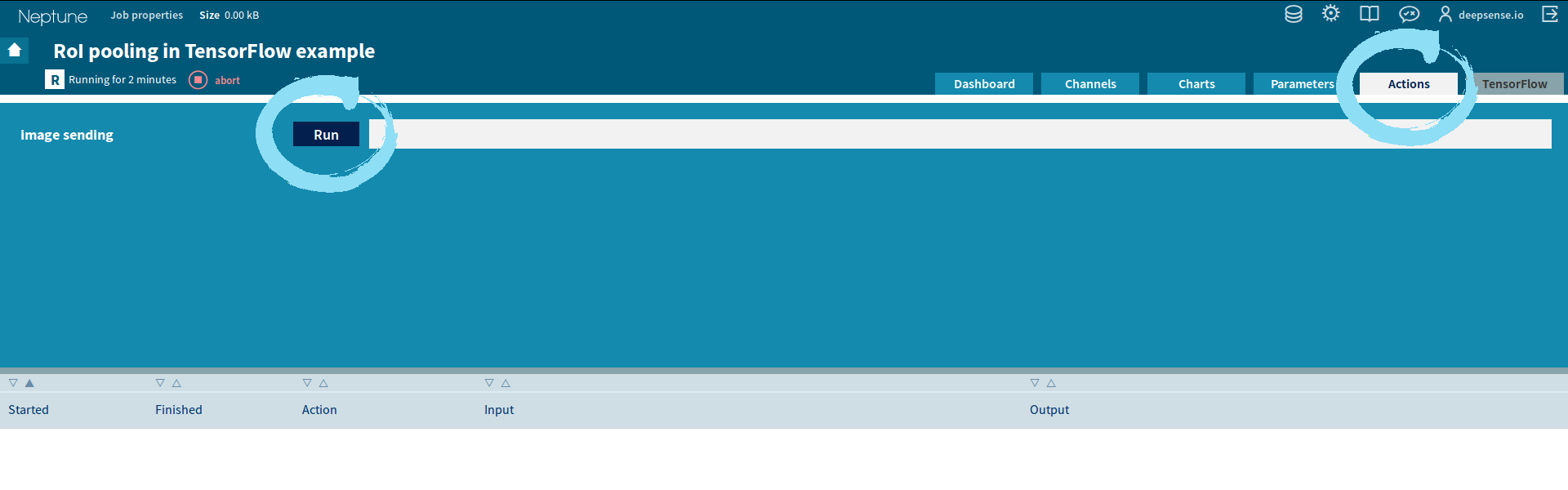
After that, we can go to the Channels tab and expand the channels ‘region proposals for RoI pooling’ and ‘network detections’ by clicking ‘+’ signs.
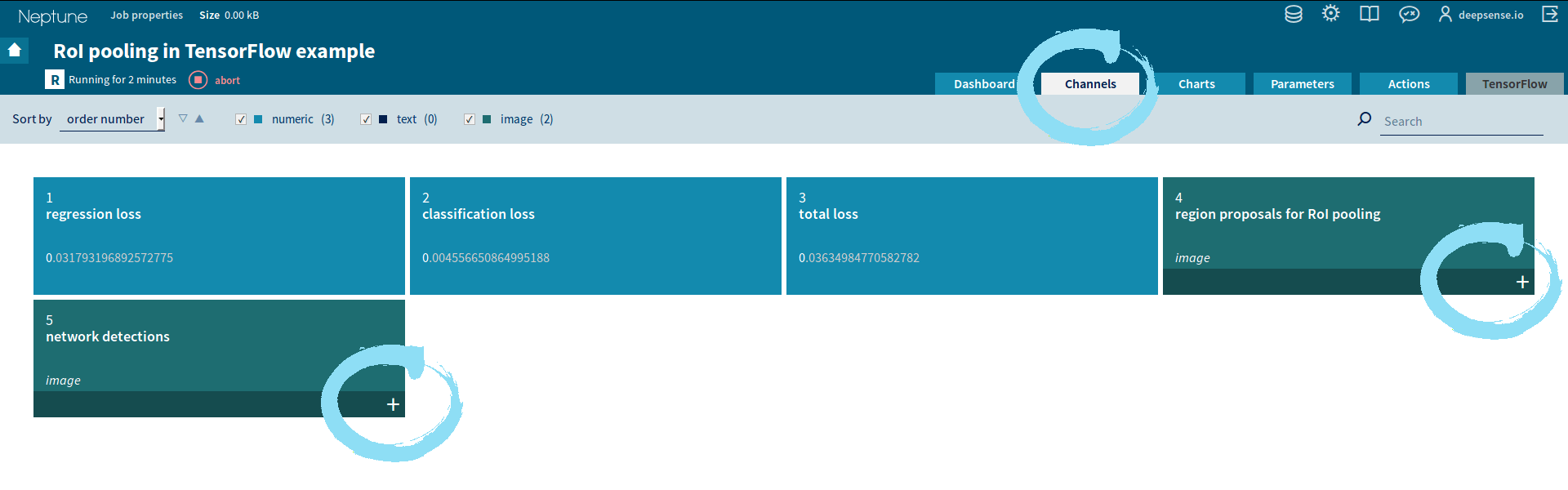
Now we can see the RoIs in real time!
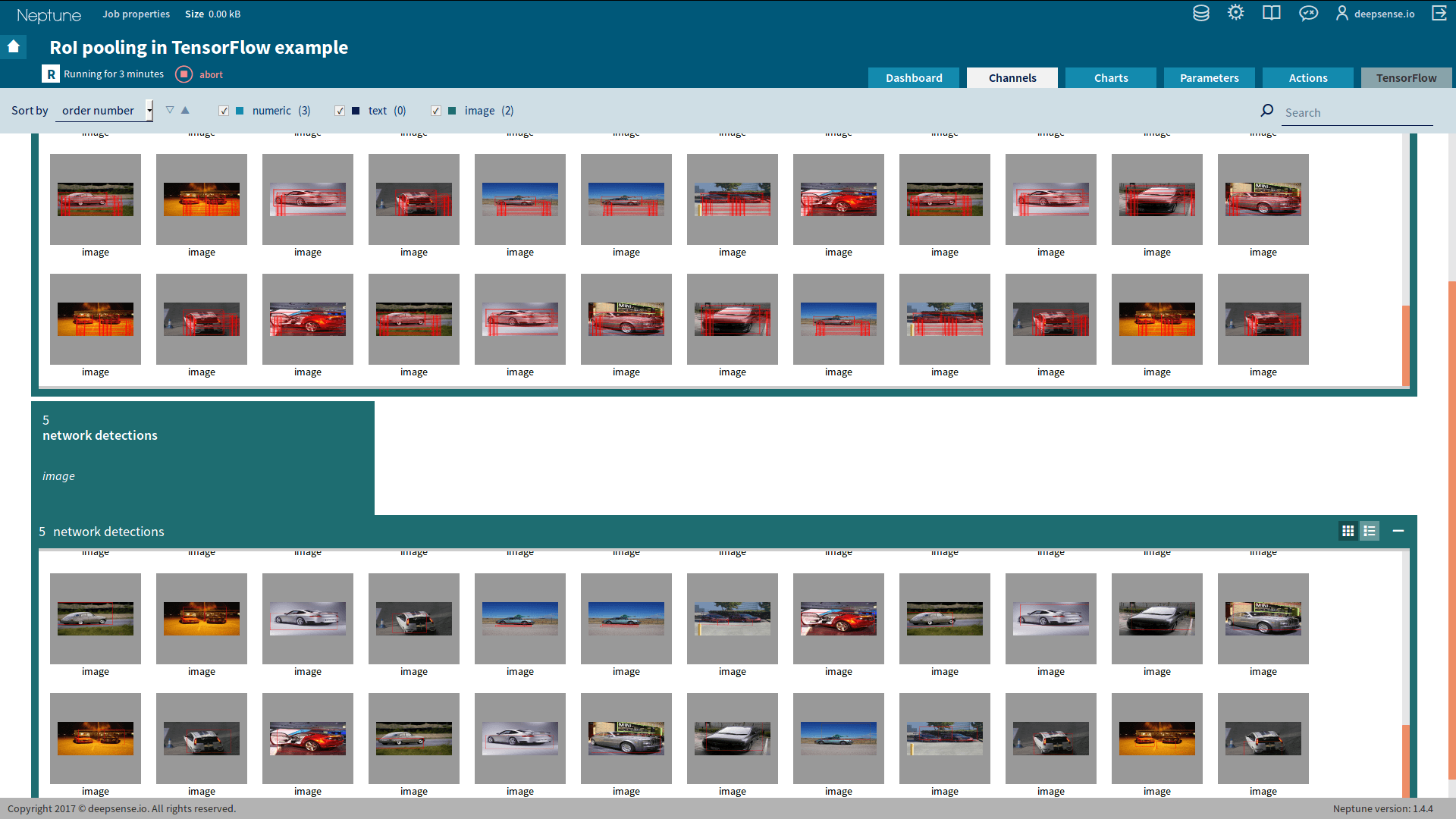
We can click on the pictures to zoom them. If we want Neptune to stop sending new images, we go to the Actions tab and click ‘RUN’ again.
An exemplary NeptuneGo execution of our script can be found here.
Summary
We hope you enjoy our example of RoI pooling in TensorFlow and experiment managing features offered by Neptune. If you want to comment our work, don’t be hesitate to leave us feedback!
References
- R. Girshick, Fast R-CNN, IEEE International Conference on Computer Vision (ICCV), 2015.
- S. Ren, K. He, R. Girshick & J. Sun, Faster R-CNN: towards real-time object detection with Region Proposal Networks, Neural Information Processing Systems (NIPS), 2015.
- deepsense.ai, Region of interest pooling explained, 2017.