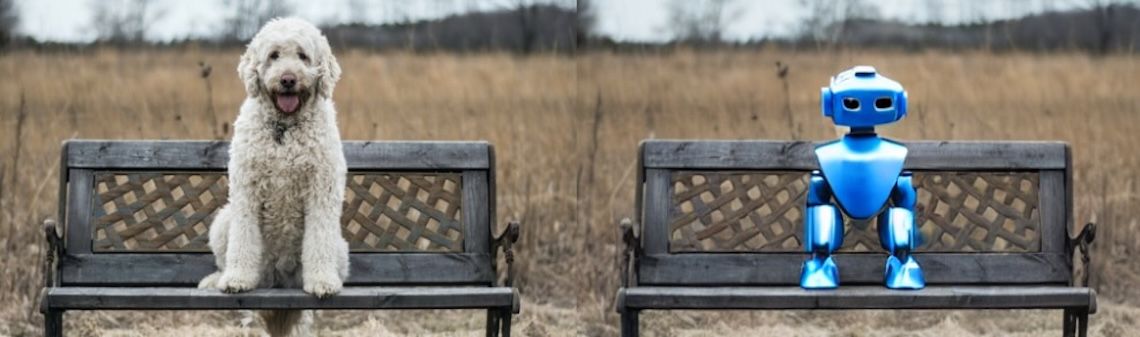
Data generation with diffusion models. Part 3: Generating custom data in the blink of an eye
Explore techniques like Stable Diffusion Inpainting, ControlNet, & GLIGEN for data generation. Beneficial for business projects yet requiring retraining.
This author has yet to write their bio.Meanwhile lets just say that we are proud Natalia Czerep contributed a whooping 3 entries.
Explore techniques like Stable Diffusion Inpainting, ControlNet, & GLIGEN for data generation. Beneficial for business projects yet requiring retraining.
One of the most challenging tasks in data generation with diffusion models is generating labels intended for semantic segmentation. At deepsense.ai, we have embraced the challenge of devising a novel approach that simultaneously generates images complete with precise segmentation masks. We are sharing the results of our work in this blog post.
It is widely known that computer vision models require large amounts of data to perform well. Unfortunately, in many business cases we are left with a small amount of data. There are several approaches to overcoming the issue of insufficient data, one of which is supplementing the available dataset with new images, which is discussed in this article.
United States of America
Poland